Have you ever wondered what exactly is machine learning? And how is it different from artificial intelligence? In this article, we will delve into the world of machine learning and artificial intelligence, exploring their definitions, differences, and applications.
Contents
- 1 Understanding Artificial Intelligence (AI)
- 2 Exploring Machine Learning (ML)
- 3 Differences Between AI and ML
- 4 Conclusion
- 5 FAQ
- 5.1 What is the difference between machine learning and artificial intelligence?
- 5.2 How is artificial intelligence defined?
- 5.3 What is machine learning?
- 5.4 What are some concepts related to machine learning?
- 5.5 How does machine learning compare to artificial intelligence?
- 5.6 What are some applications of artificial intelligence in the manufacturing industry?
- 5.7 How is artificial intelligence applied in the banking sector?
- 5.8 What are some applications of artificial intelligence in healthcare?
- 6 Source Links
Key Takeaways:
- Artificial intelligence (AI) and machine learning (ML) are distinct concepts, with ML being a subset of AI.
- AI involves computers emulating human thought and performing tasks, while ML enables systems to identify patterns, make decisions, and improve through experience and data.
- AI has a broader scope of applications, while ML focuses on algorithms learning from data to perform specific tasks.
- Both AI and ML have various applications across industries such as manufacturing, banking, and healthcare.
- Understanding the distinctions and potential of AI and ML is essential in leveraging their capabilities in the digital age.
Understanding Artificial Intelligence (AI)
Artificial intelligence (AI) is an exciting field that focuses on developing computers and robots that can mimic human behavior and intelligence. AI involves tasks such as analyzing data, contextualizing information, and automating processes to perform complex tasks.
AI technologies encompass a range of capabilities, including natural language processing, which enables computers to understand and communicate with humans using human language. This technology is used in applications like virtual assistants and chatbots.
AI vs ML: What’s the Difference?
AI and machine learning (ML) are often used interchangeably, but they are distinct concepts. AI is the broader concept of enabling machines to exhibit human-like behavior and cognition. ML, on the other hand, is a subset of AI that focuses on using algorithms and statistical models to analyze data, learn insights, and make predictions.
Machine learning is an important component of AI, as it provides the ability for systems to learn from experience and improve their performance over time. This is achieved through training models on large datasets to identify patterns and make accurate predictions. Deep learning, a subfield of ML, utilizes neural networks to learn complex patterns and enhance the capabilities of AI systems.
AI has diverse applications across various industries. In manufacturing, AI is used for quality control, predictive maintenance, and optimizing energy consumption. In the banking sector, AI powers fraud detection systems, automates customer service, and ensures secure biometric authentication.
Furthermore, AI is revolutionizing the healthcare industry by enabling clinical decision support systems, predicting patient outcomes, and improving provider-patient interactions through natural language processing.
As AI continues to advance, it is driving innovation and transforming numerous sectors, making our lives more efficient, convenient, and secure. Understanding AI and its applications is crucial in today’s digital age as we navigate the ever-expanding possibilities of artificial intelligence.
Exploring Machine Learning (ML)
Machine learning is a fundamental concept in the field of artificial intelligence (AI). It involves the use of algorithms to enable systems to learn from data, identify patterns, and make informed decisions. By continuously improving their performance over time, machine learning models have far-reaching applications across various industries.
One notable method of machine learning is deep learning, which leverages neural networks to independently learn complex patterns and representations. Deep learning algorithms have brought significant advancements in areas such as computer vision, natural language processing, and speech recognition.
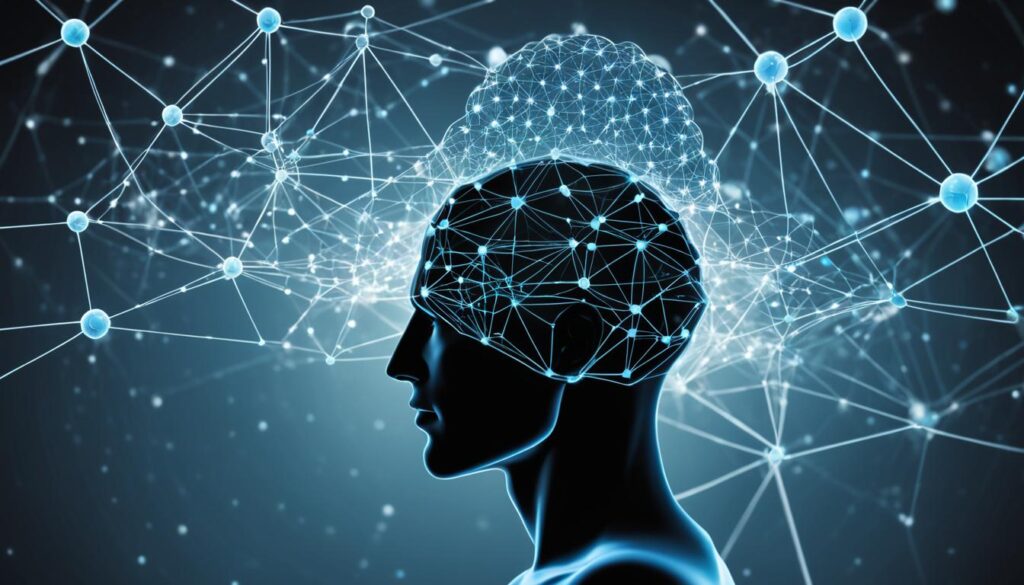
The Power of Machine Learning Algorithms
Machine learning algorithms serve as the backbone of ML models, enabling them to process and analyze vast amounts of data to extract meaningful insights. These algorithms can be broadly categorized into three types:
- Supervised Learning: In supervised learning, models are trained using labeled datasets, where the desired output is known. The algorithm learns to predict or classify new and unseen data based on the patterns observed during training.
- Unsupervised Learning: Unsupervised learning involves training ML models on unlabeled data. The algorithm discovers hidden patterns, structures, or relationships within the dataset without any predefined output.
- Reinforcement Learning: In reinforcement learning, models learn through interaction with their environment. They receive feedback in the form of rewards or penalties based on their actions, enabling them to optimize their performance.
Machine learning finds applications in various domains, driving innovation and improving efficiency. Let’s explore some of the common use cases:
Application | Description |
---|---|
Personalized Recommendations | Machine learning algorithms analyze user preferences and behavior to provide tailored recommendations for products, services, or content. |
Fraud Detection | ML models can detect patterns and anomalies in financial transactions, identifying potential fraudulent activities and preventing financial losses. |
Image Recognition | Deep learning algorithms excel in recognizing and categorizing objects, enabling applications such as autonomous vehicles, facial recognition, and medical imaging. |
Natural Language Processing | ML models process and understand human language, powering voice assistants, chatbots, and machine translation. |
Machine learning algorithms play a crucial role in AI advancements, enabling systems to learn from vast amounts of data and make informed decisions in real-time.
By continuously evolving and adapting to new information, machine learning models offer powerful solutions to complex problems across industries. As organizations harness the potential of machine learning, we can expect further advancements and innovations that will shape the future of artificial intelligence.
Differences Between AI and ML
While understanding machine learning and artificial intelligence may seem similar, they are distinct concepts with unique characteristics. AI, or artificial intelligence, encompasses the broader goal of enabling machines to mimic human cognition and perform a wide range of tasks. On the other hand, ML, or machine learning, is a subset of AI that specifically focuses on algorithms learning from data and using that knowledge to perform tasks.
AI has a wide scope of applications and is utilized in various industries ranging from healthcare to manufacturing. It involves tasks such as data analysis, contextualizing information, and automating processes. Technologies like natural language processing and computer vision fall under the umbrella of AI.
Meanwhile, ML has a more limited scope compared to AI. It uses statistical models and structured or semi-structured data to learn insights, patterns, and make informed decisions. Deep learning, a method of ML, uses neural networks to independently learn complex patterns. ML is commonly used in applications such as personalized recommendations and fraud detection.
AI vs ML: While AI aims to mimic human cognition, ML focuses on learning from data to perform tasks.
To further illustrate the differences between AI and ML, let’s take a closer look at their characteristics:
AI | ML |
---|---|
Enables machines to mimic human cognition | Focuses on algorithms learning from data |
Wide scope of applications | More limited scope |
Works with all types of data | Uses statistical models and structured/semi-structured data |
While AI and ML are interconnected, understanding their distinctions is essential when it comes to leveraging their capabilities in today’s digital landscape.
In the manufacturing industry, AI plays a crucial role in enhancing productivity, improving efficiency, and minimizing operational costs. Some of the prominent applications include:
- Equipment Error Detection: AI-powered systems can analyze sensor readings and detect anomalies, enabling proactive maintenance and reducing downtime.
- Predictive Maintenance: ML algorithms can predict equipment failures based on data patterns, allowing manufacturers to schedule maintenance before problems occur.
- Energy Consumption Optimization: AI algorithms can optimize energy usage by analyzing data from sensors and adjusting operational parameters accordingly.
The integration of AI and ML in manufacturing is transforming traditional processes, enabling smart factories and industry 4.0 initiatives.
AI Applications in Banking
In the banking sector, AI has reshaped the way financial institutions operate, providing enhanced customer experiences and robust fraud detection capabilities. Here are some notable applications:
- Fraud Detection: AI algorithms can analyze large volumes of data to identify patterns indicative of fraudulent activities, helping banks safeguard their customers’ assets.
- Biometrics: AI-powered biometric authentication systems enable secure and seamless verification through methods such as facial recognition and fingerprint scanning.
- Customer Service Automation: Chatbots and virtual assistants equipped with natural language processing capabilities allow banks to automate routine customer inquiries, providing instant and efficient support.
The adoption of AI in banking is paving the way for personalized services, improved risk management, and streamlined operations.
AI Applications in Healthcare
In the healthcare industry, AI and ML have the potential to revolutionize patient care, clinical decision-making, and disease diagnosis. The following applications showcase the transformative power of these technologies:
Application | Description |
---|---|
Clinical Decision Support | AI algorithms assist healthcare professionals in making accurate diagnoses, selecting appropriate treatment options, and predicting patient outcomes. |
Outcome Prediction | ML models analyze patient data to predict disease progression, treatment effectiveness, and individual health outcomes. |
Provider-Patient Interaction Analysis | AI-powered tools evaluate patient-provider interactions, identifying communication gaps and potential areas for improvement. |
The integration of AI and ML in healthcare has the potential to enhance patient care, reduce medical errors, and enable personalized treatment plans.
As technology continues to advance, AI and ML will continue to revolutionize various industries, offering endless possibilities for optimization, automation, and innovation.
Conclusion
In conclusion, machine learning and artificial intelligence are two related concepts that are often used interchangeably but have distinct differences. Machine learning, as a subset of artificial intelligence, focuses on the ability of systems to learn from data and make decisions. On the other hand, artificial intelligence goes beyond machine learning and encompasses a broader range of tasks that involve emulating human cognition.
Both machine learning and artificial intelligence have significant applications and are transforming various industries. Machine learning is widely used in personalized recommendations, fraud detection, and other areas where pattern recognition and decision-making based on data are crucial. Artificial intelligence, with its ability to perform tasks like analyzing data, contextualizing information, and automating processes, finds applications in manufacturing, banking, healthcare, and other sectors.
To fully leverage the potential of machine learning and artificial intelligence, it is essential to understand their distinctions and capabilities. Machine learning leverages data-driven insights to improve decision-making, whereas artificial intelligence encompasses a broader range of tasks and includes machine learning as a component. As the digital age progresses, the applications and impact of both machine learning and artificial intelligence are poised to grow, making it imperative for industries and individuals to grasp their potential and embrace their transformative power.
FAQ
What is the difference between machine learning and artificial intelligence?
Machine learning is a subset of artificial intelligence. While artificial intelligence refers to computers emulating human thought and performing tasks, machine learning specifically focuses on using algorithms to learn from data and improve performance over time.
How is artificial intelligence defined?
Artificial intelligence (AI) involves the development of computers and robots that can behave like humans. It encompasses tasks such as analyzing data, contextualizing information, and automating processes.
What is machine learning?
Machine learning (ML) is a pathway to artificial intelligence. It uses algorithms to learn insights and patterns from data, enabling systems to make decisions and improve performance through experience.
Machine learning encompasses concepts such as deep learning, which uses neural networks to learn complex patterns independently, and statistical models to analyze structured and semi-structured data.
How does machine learning compare to artificial intelligence?
Machine learning is a more specific subset of artificial intelligence. While machine learning focuses on algorithms learning from data, artificial intelligence has a broader scope, including tasks such as natural language processing and computer vision.
What are some applications of artificial intelligence in the manufacturing industry?
Artificial intelligence is used in manufacturing for tasks such as equipment error detection, predictive maintenance, and optimizing energy consumption.
How is artificial intelligence applied in the banking sector?
Artificial intelligence has various applications in banking, including fraud detection, biometrics, and automating customer service processes.
What are some applications of artificial intelligence in healthcare?
In healthcare, artificial intelligence is used for tasks such as clinical decision support, outcome prediction, and analyzing provider-patient interactions.